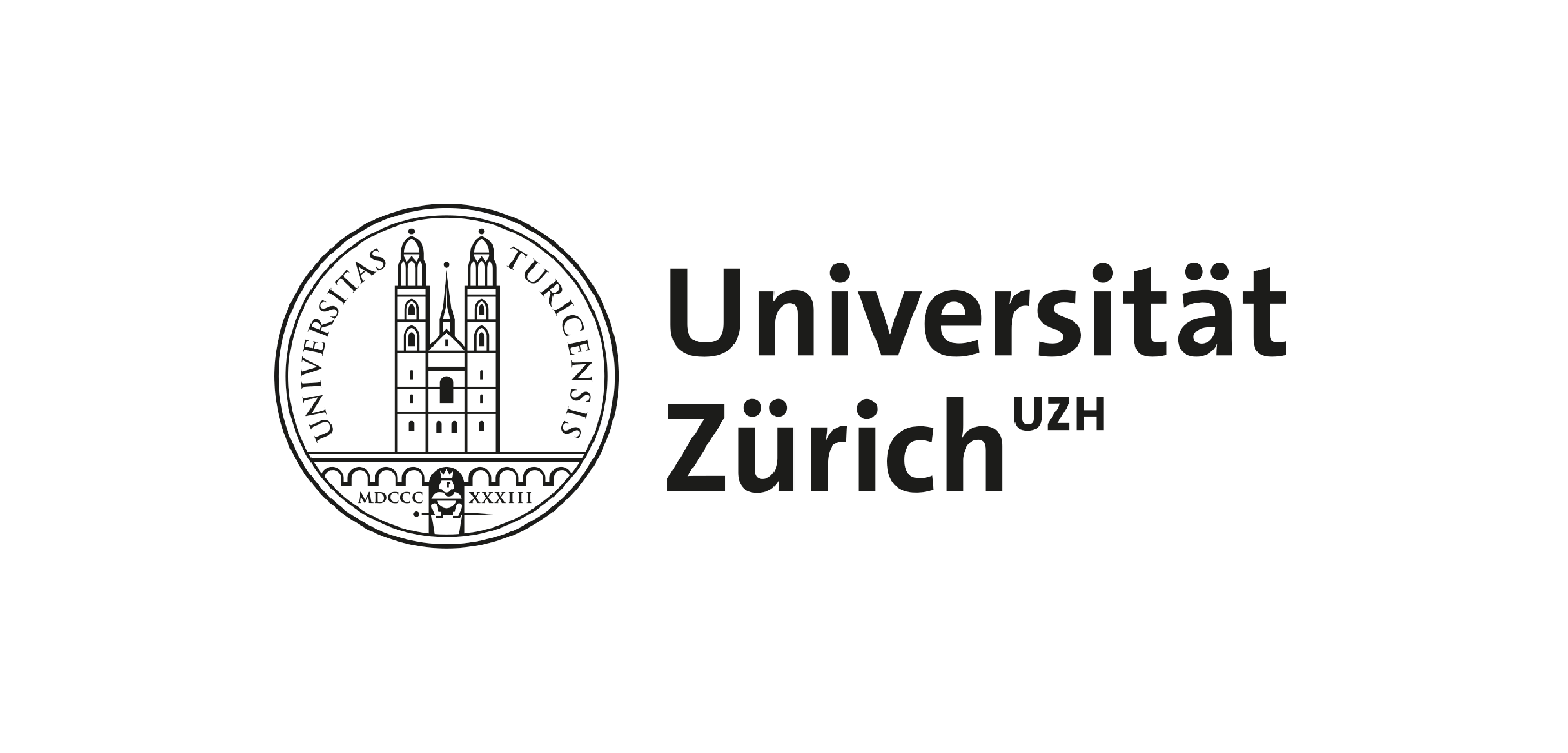
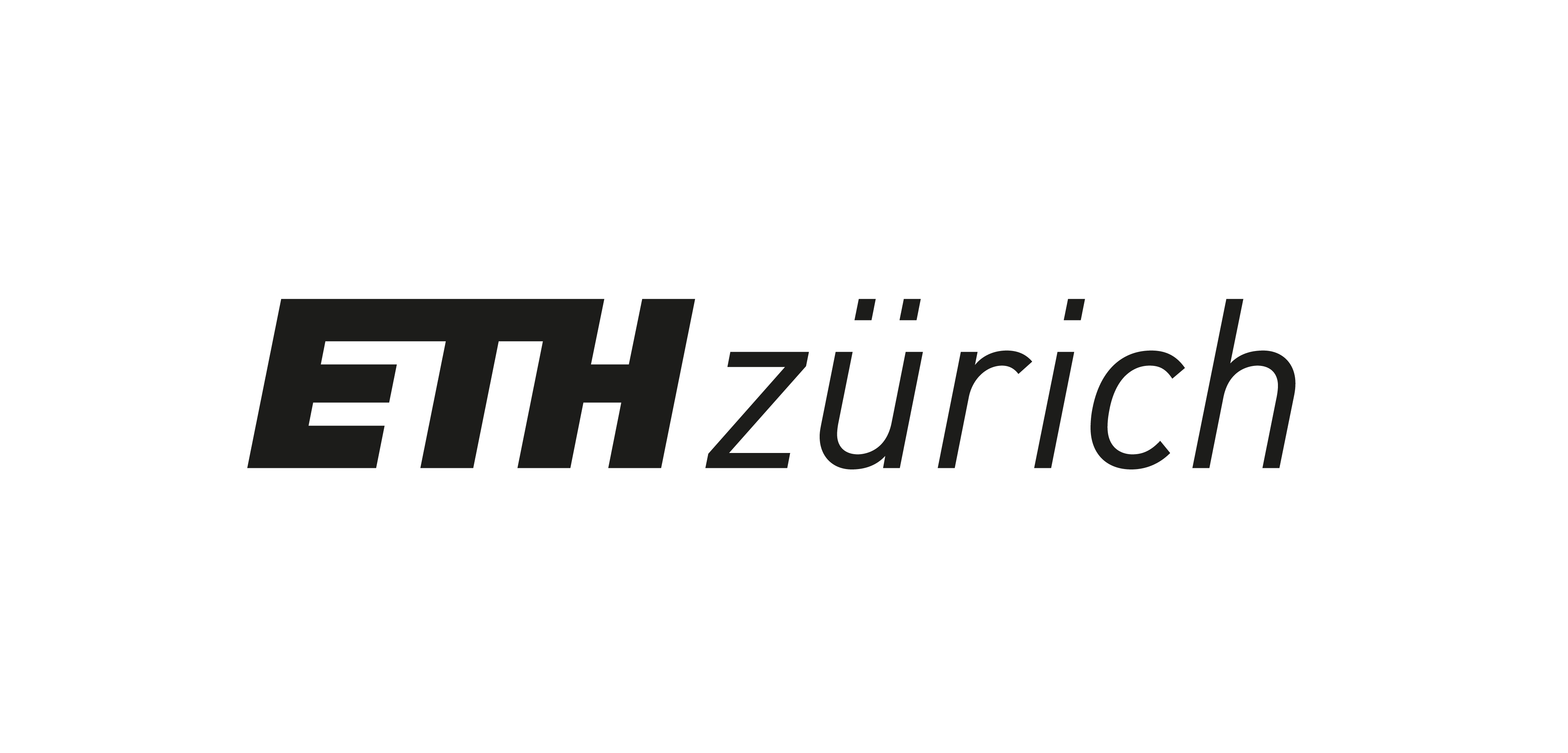
To stay up-to-date with the lab, don't forget to follow us on Twitter (@BodenmillerLab)!
News
- Bernd was invited to the Strategy & Insider podcast to talk precision oncology, AI and the future of diagnostics.May 30
Alongside Prof. Dr. Andreas Wicki, Bernd shared his vision to transform cancer diagnosis, treatment, and management. Click the link below to listen to his take on the future of diagnostics.
- We're pleased to announce two new Ph.D. students, Quentin Hellier and Simone Häfliger. Welcome on board!Jan 8
- Huge congratulations to Tsuyoshi for successfully defending his PhD!Oct 26
The subject of his Thesis is: "Highly-Multiplexed and Sensitive Antibody-Based Imaging with DNA Barcodes and Metal Isotope-Labeling".
- A big welcome to our newest post-doctoral fellow, Genki Usui! We're delighted to have you join our team.Oct 2
- Today, the Bodenmiller lab celebrated its 10 year anniversary. Thanks to all our alumni who travelled far and wide to join us!Sep 8
- Welcome to our newest team members, Lydia Schulla and Egle Ervin. We're thrilled to have them on board!Sep 1
- Congratulations to Lena for successfully defending her PhD!Jul 13
The title of her Thesis is: Single-Cell Analysis of Cancer-Associated Fibroblast Heterogeneity with a Focus on
Spatial Distribution and Clinical Implications. Lena's PhD was awarded with a Distinction.
To stay up-to-date with the lab, don't forget to follow us on Twitter (@BodenmillerLab)!
Research
Methods development
We develop experimental and computational methods to study tumor ecosystems on the single-cell level. In such an ecosystem, many cell types, including tumor, stromal, immune and endothelial cells, interact and communicate in multicellular assemblies. We seek to understand how tumor ecosystems function and ultimately how their properties affect disease. We generate comprehensive single-cell datasets of tumors from many patients, which requires detection of dozens of markers simultaneously. We have pioneered mass cytometry-based methods for simultaneous multiplexed marker detection and analysis in both dissociated tissues and on tissue sections, and continue to develop these methods further (see technology section).
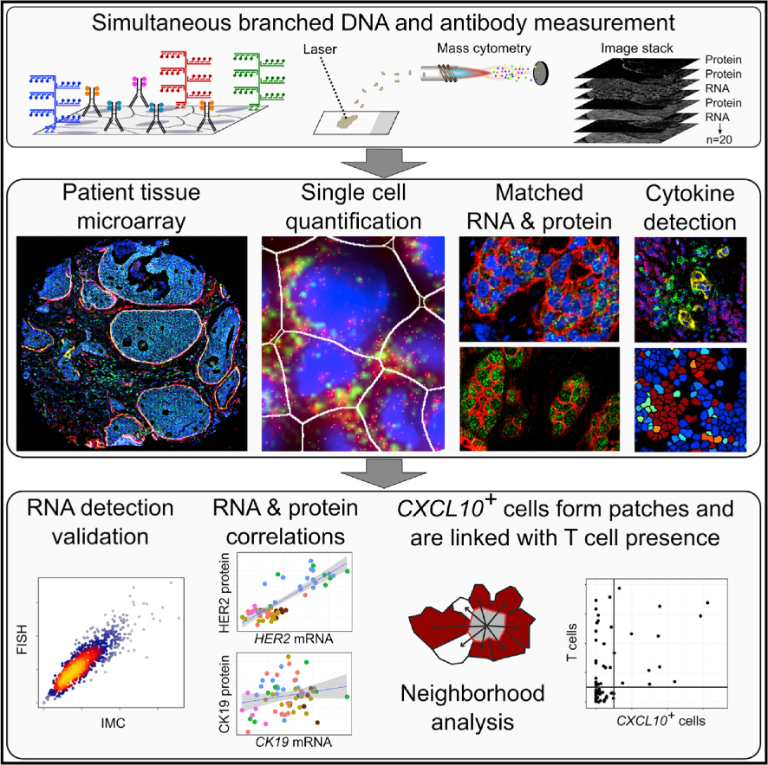
Selected Publications
Translational research and precision medicine
Solid tumors are multicellular ecosystems of diverse cell types that interact to manifest emergent phenotypes which ultimately determine clinical outcome. We combine suspension or imaging mass cytometry with computational techniques to systematically describe cell phenotypes in tumor ecosystems and to examine their distribution across patients and tumor types. We generate atlases of human tumors from large patient cohorts with known clinical outcome, and identify cellular and spatial phenotypes associated with disease progression. These atlases lay the foundation for improved patient stratification and provide potential drug targets for ongoing study. These data are also the foundation for follow-up studies to understand mechanisms of the disease. We are part of several large-scale, multi-center projects bringing together clinicians, research labs and pharmaceutical companies, in which we develop mass cytometry and imaging mass cytometry for precision medicince applications.
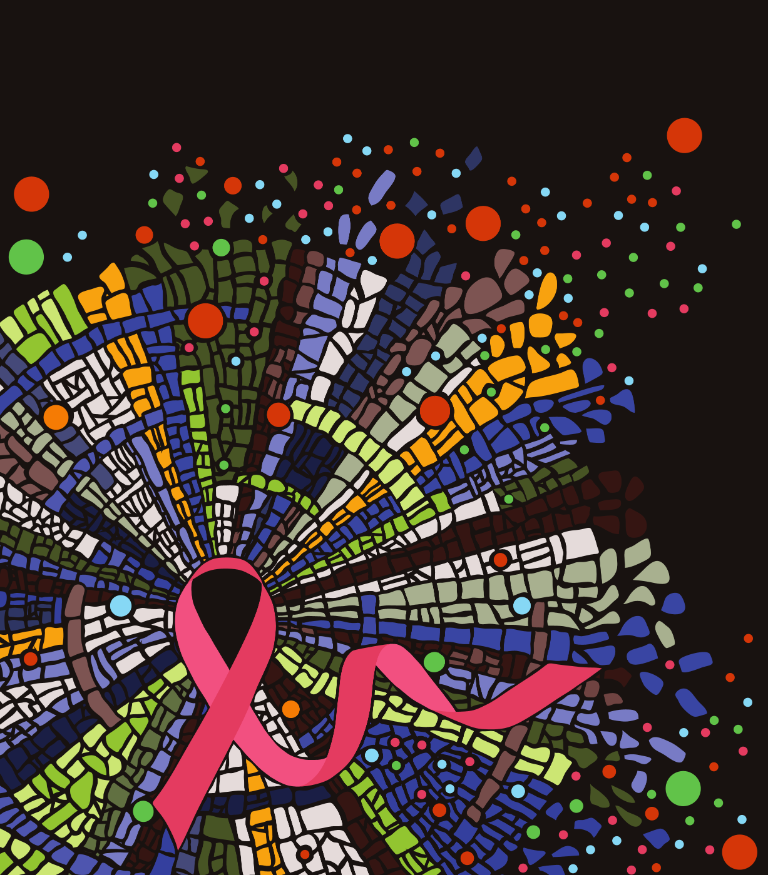
Selected Publications
Mechanisms of cancer
Single-cell systems biology analyses of tumor samples yield a wealth of data about cancer biology. To understand the regulatory networks underlying the disease, we use algorithmic and data-driven approaches to model subpopulations of cells and their signaling network structures. Further, using data from imaging mass cytometry, we model how signaling network states spatially couple with those of other cells. As a complement to modeling and associative studies, we use in vitro patient-derived organoid and cell co-culture models to conduct small molecule screens and carry out perturbation studies aimed at understanding mechanistic aspects of tumor biology.
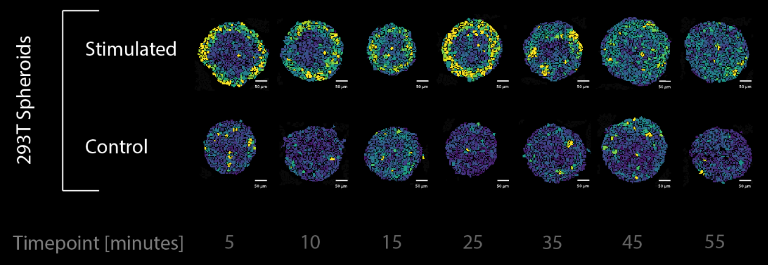
Selected Publications
The Bodenmiller lab is supported by these consortia:
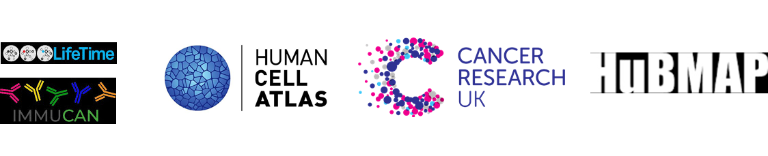
Technology
Imaging Mass Cytometry
Single-cell systems biology of cancer requires methods to measure multiple markers within tumors, quantitatively, and with spatial and single-cell resolution. Based on our earlier work on suspension mass cytometry, the Bodenmiller group has pioneered a spatial mass spectrometric approach called imaging mass cytometry (IMC) for the simultaneous and spatially-resolved quantification of approximately 50 markers on single cells. We employ IMC-based methods to study the cellular composition, spatial organization and regulation of tissue ecosystems, for insights into health and disease.
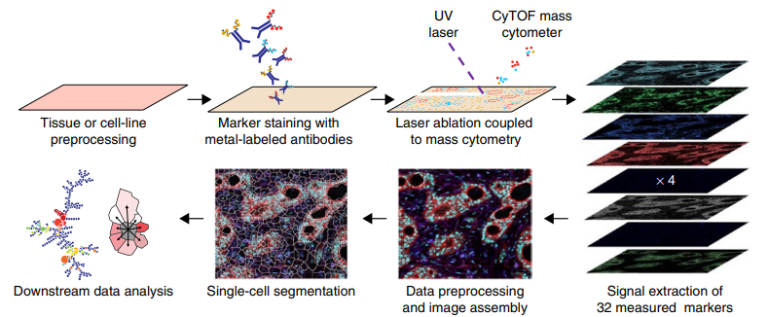
Imaging mass cytometry: measurement
In mass cytometry, we use a mass spectrometer to measure protein and/or transcript levels within cells, using antibodies or RNA probes linked to different metal isotopes. In imaging mass cytometry, we have extended this technology to solid tissue samples such as tumor biopsies, analyzing them spatially and capturing effects of the local microenvironment on tumor cells. We apply IMC in 2D and are also developing it in 3D. Mass cytometry can in principle reliably differentiate over a 100 probes. We are continuously improving the speed, number of markers, resolution, reliability, sensitivity, biological interpretability and overall quality of high-dimensional single cell analysis.
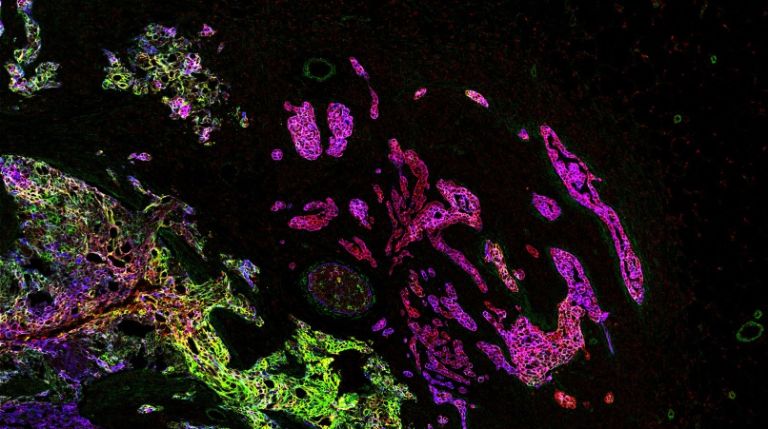
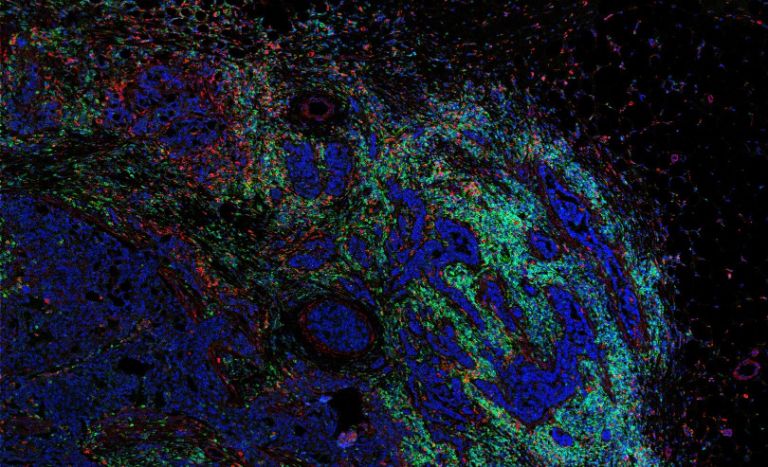
Imaging mass cytometry: analysis
Deriving relevant biological information from high-dimensional datasets is an ongoing challenge in systems biology. We explore the capabilities of existing statistical and image analysis tools to analyse imaging mass cytometry data in a meaningful way. We also develop software to process, visualize and analyze high dimensional IMC datasets.
Software
The Bodenmiller GitHub page has code and scripts for many projects.
The IMC workflow provides an overview on IMC data analysis approaches.
histoCAT


histoCAT is an open-source visualization and analysis toolbox for exploration of rich multidimensional IMC datasets. It enables parallel visualization of images and single cell phenotypic distributions and includes methods to identify and quantify cell-cell interactions within tissue. Read the paper.
histoCAT++


histoCAT++ is a later implementation with more advanced features. Read the paper.
steinbock
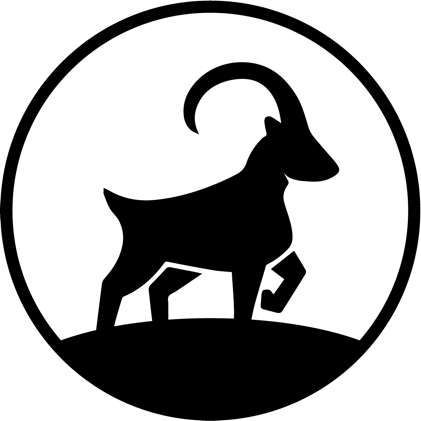
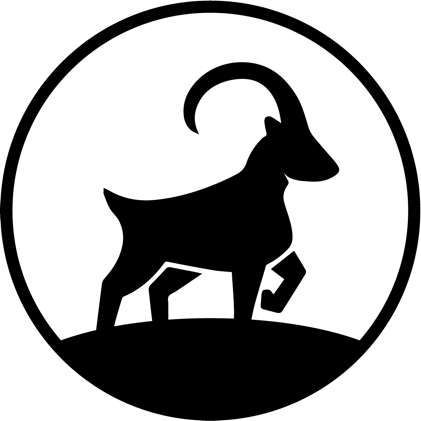
steinbock is a collection of tools for multi-channel image processing using the command-line or Python code. Supported tasks include IMC data preprocessing, supervised multi-channel image segmentation, object quantification and data export to a variety of file formats. The steinbock framework is fully documented, integrates well with downstream analysis packages and is available as platform-independent Docker container, ensuring reproducibility.
cytomapper
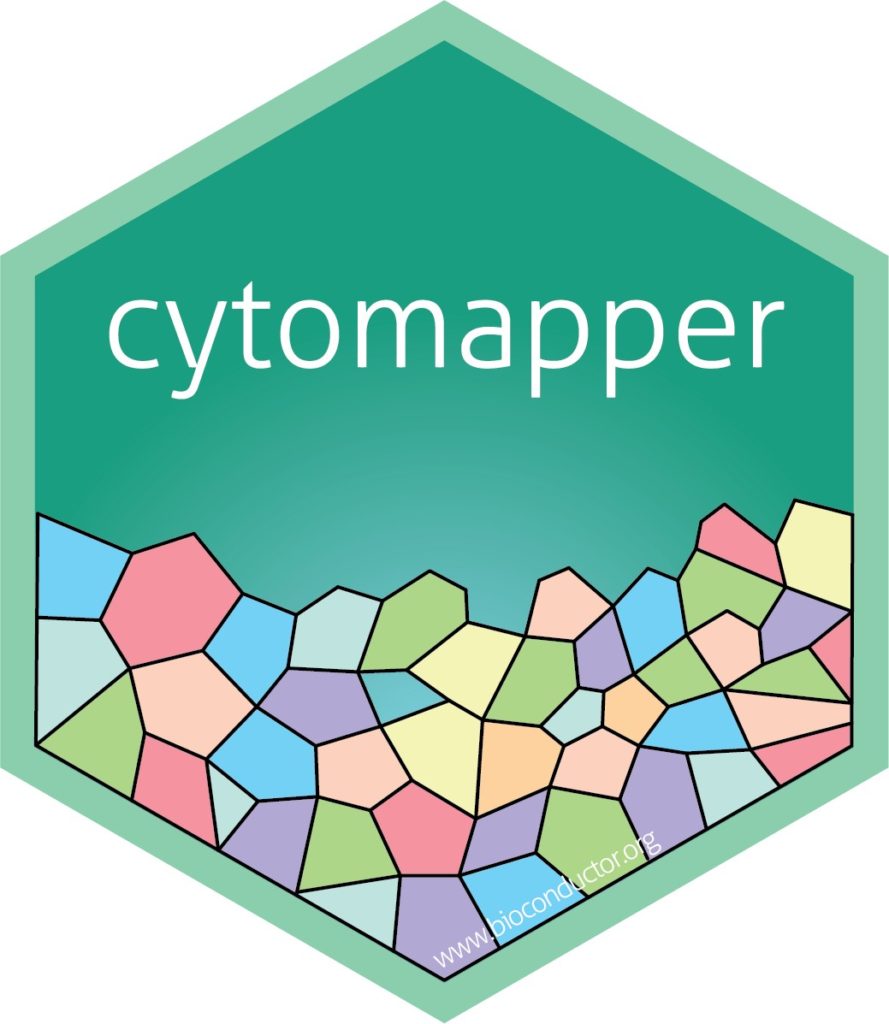
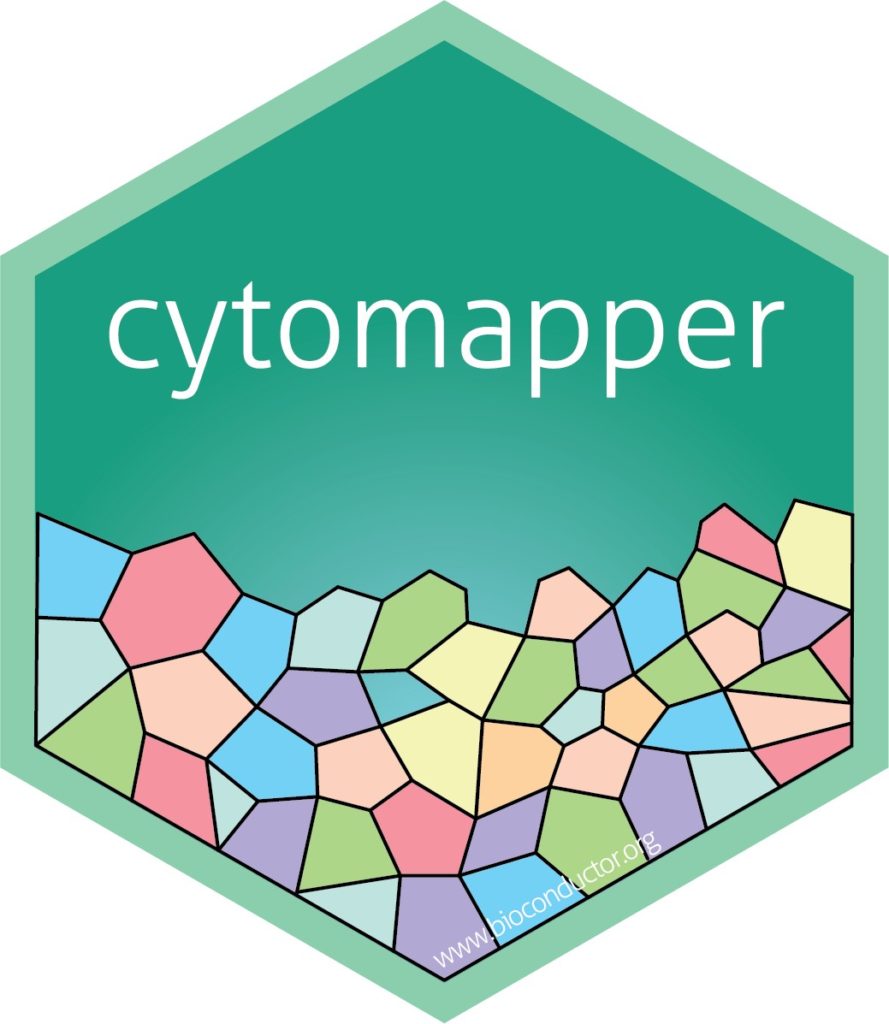
Multiplex imaging cytometry acquires spatially-resolved single-cell expression values of selected proteins in a sample. Cytomapper can be used to visualize the multiplexed read-outs obtained with this technique. The main functions of this package allow (i) the visualization of pixel-level information across multiple channels and (ii) the display of cell-level information (expression and/or metadata) on segmentation masks.
imcRtools
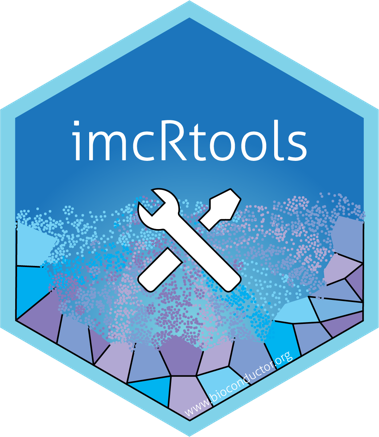
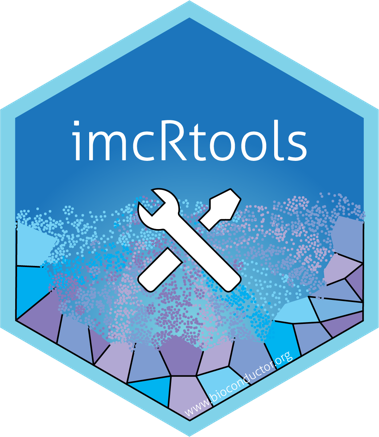
The imcRtools R/Bioconductor package supports the handling and analysis of imaging mass cytometry and other highly multiplexed imaging data. The main functionality includes reading in single-cell data after image segmentation and measurement, data formatting to perform channel spillover correction and a number of spatial analysis approaches.
AirLab
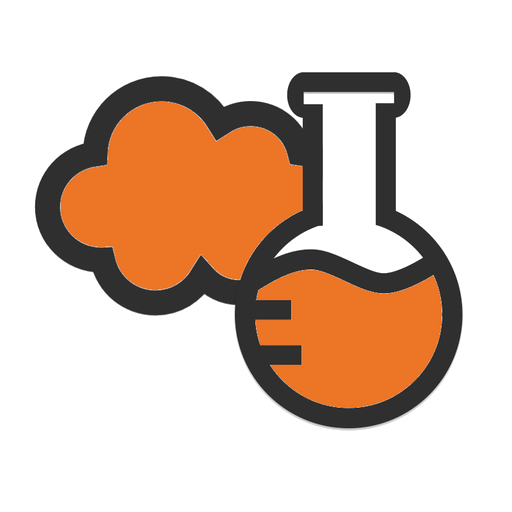
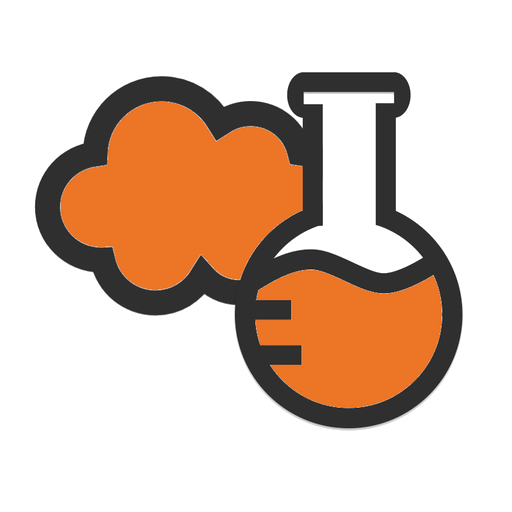
AirLab is a cloud-based laboratory-management tool for antibody-based research. You can use it to manage antibody stocks, antibody panels for CyTOF and Helios, and antibody-based experiments and results. Read the paper.
CellCycleTRACER
CellCycleTRACER permits correction of mass cytometry data for confounding effects of cell cycle and volume, as long as 4 measurement channels are left free for the relevant markers. Read the paper here.
Adnet
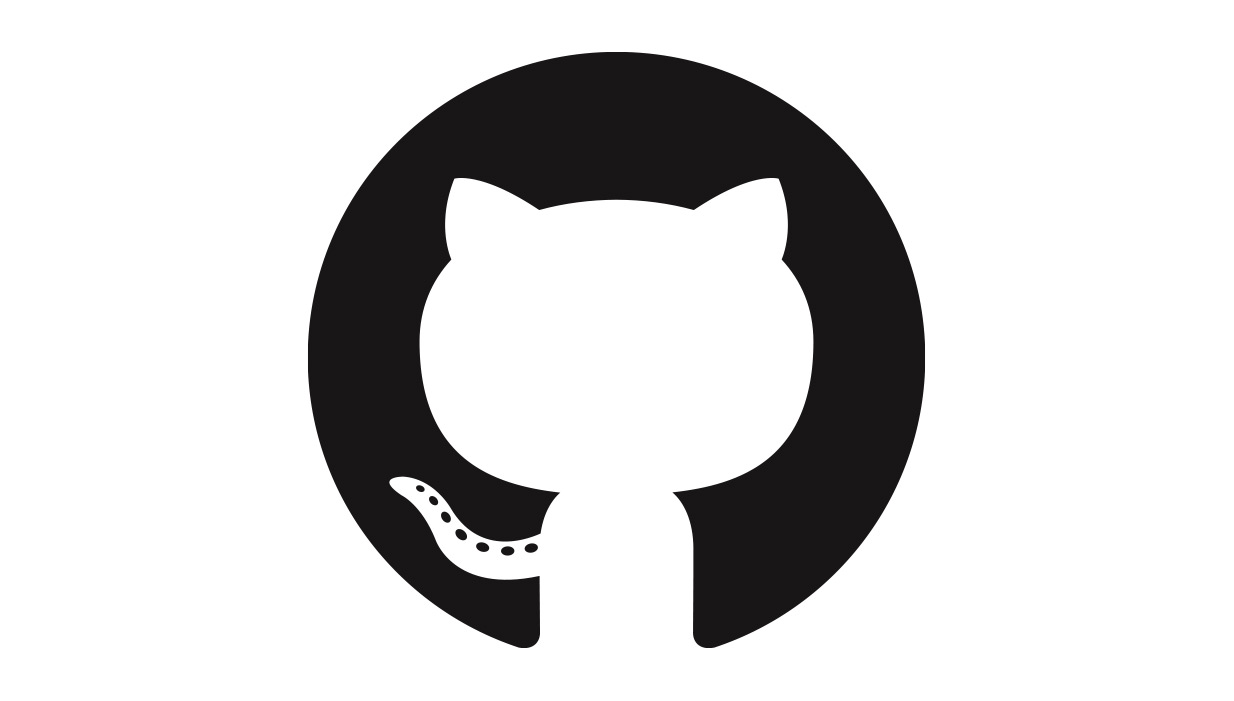
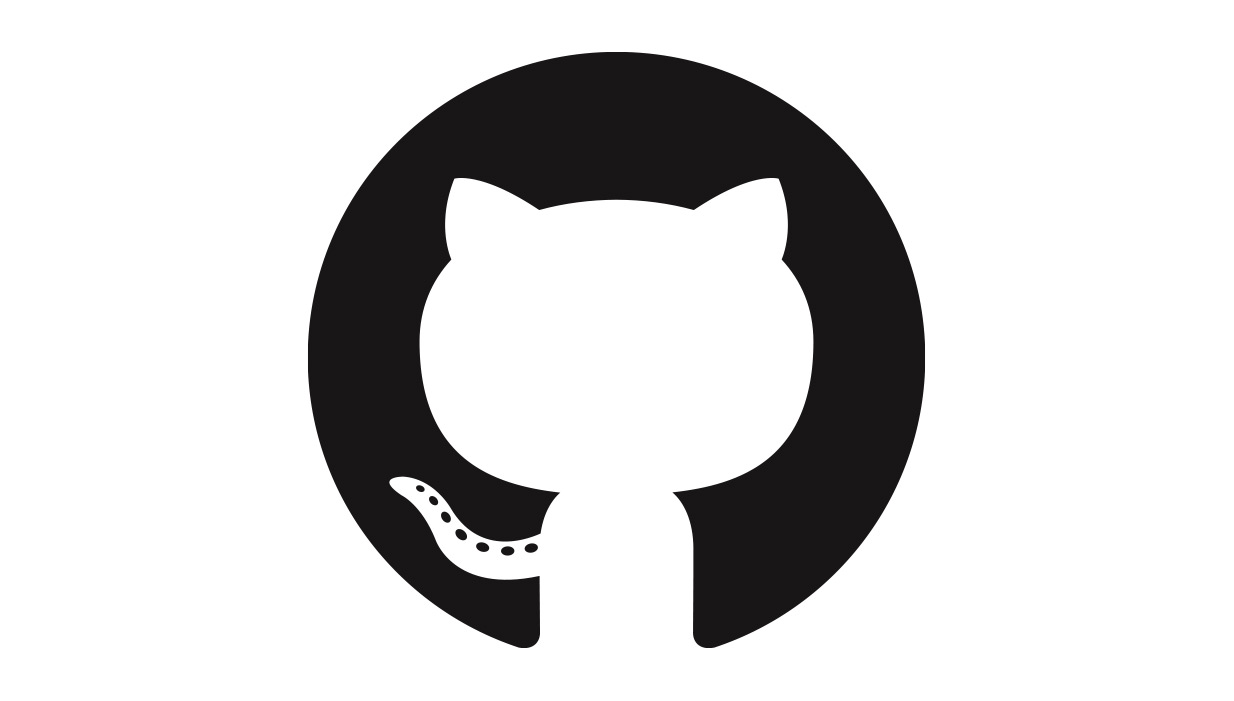
Adnet is a set of analysis scripts used for the paper “Influence of node abundance on signaling network state and dynamics analyzed by mass cytometry” by XK Lun et al. Read the paper here.
Protocols
The Bodenmiller lab protocols page is in progress.
People
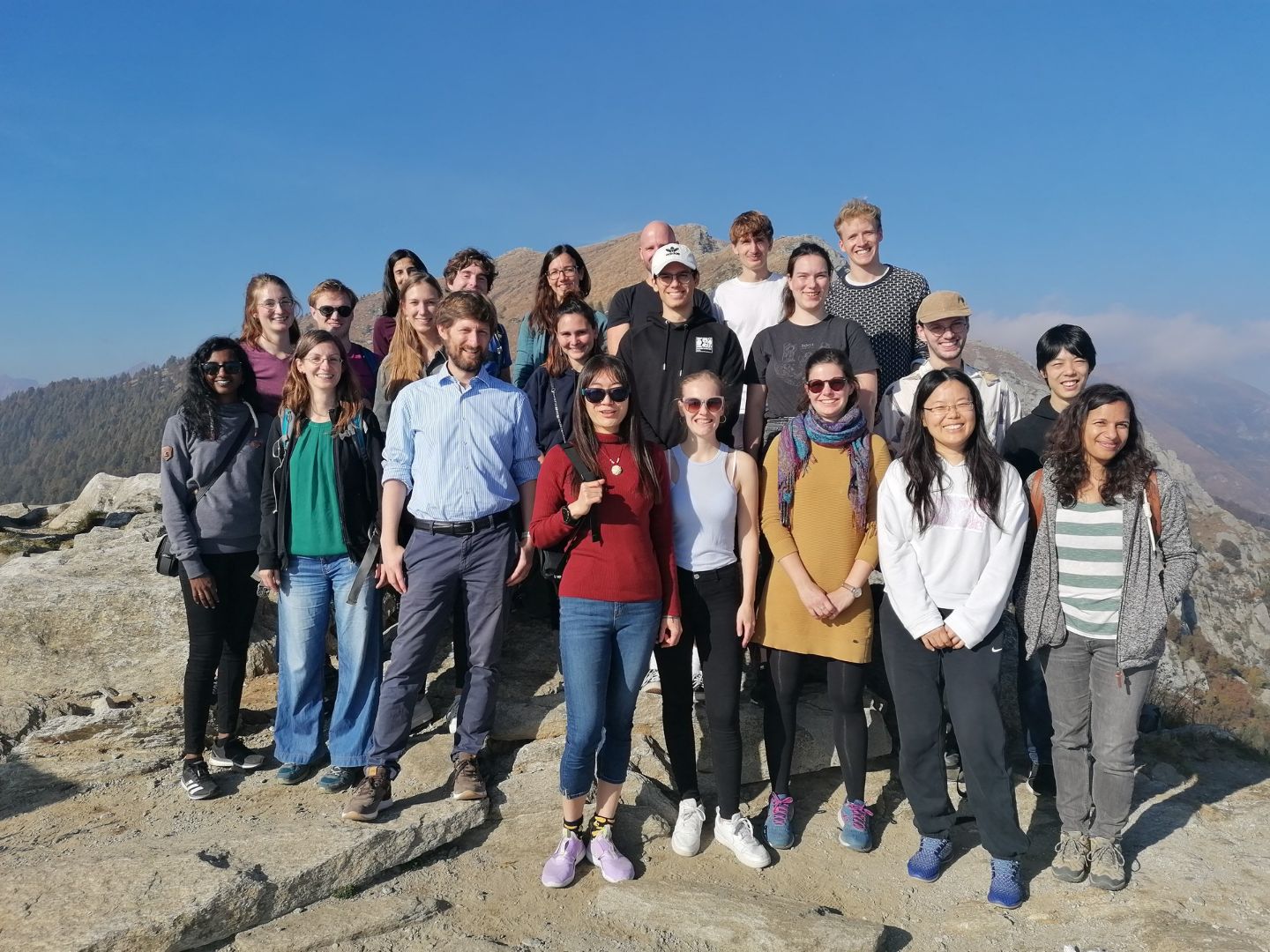
Current Members
Prof. Dr.
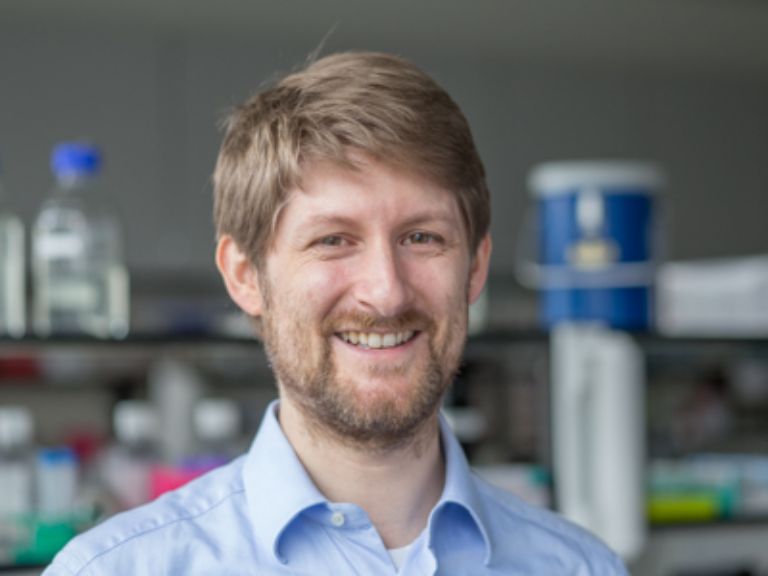
Single-cell systems biology of cancer requires methods to measure multiple markers within tumors, quantitatively and at single-cell resolution. To this end, the Bodenmiller group has pioneered a single-cell mass spectrometric approach called mass cytometry (CyTOFTM). This technology allows the simultaneous and high-throughput quantification of approximately 50 markers, including proteins and their modifications, on single cells. We employ mass cytometry-based methods to study the cellular composition and regulation of tissue ecosystems, for insights into health and disease.
Laboratory Manager
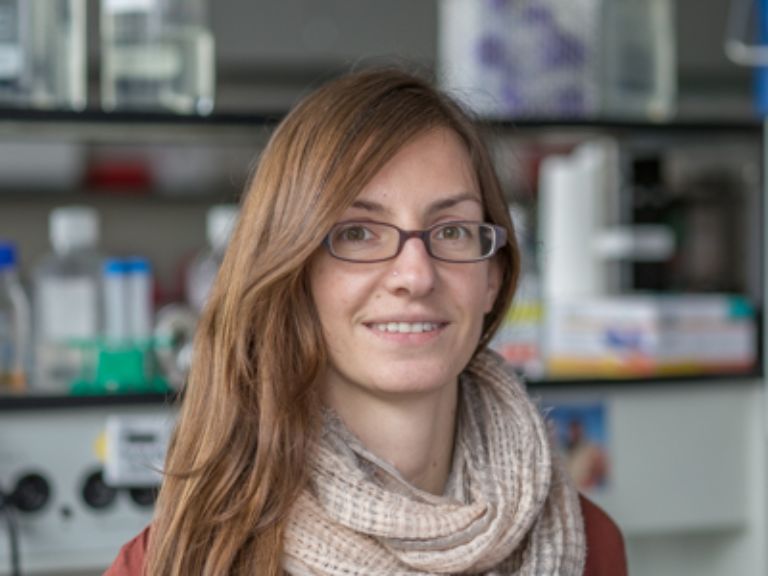
Senior Research Associate
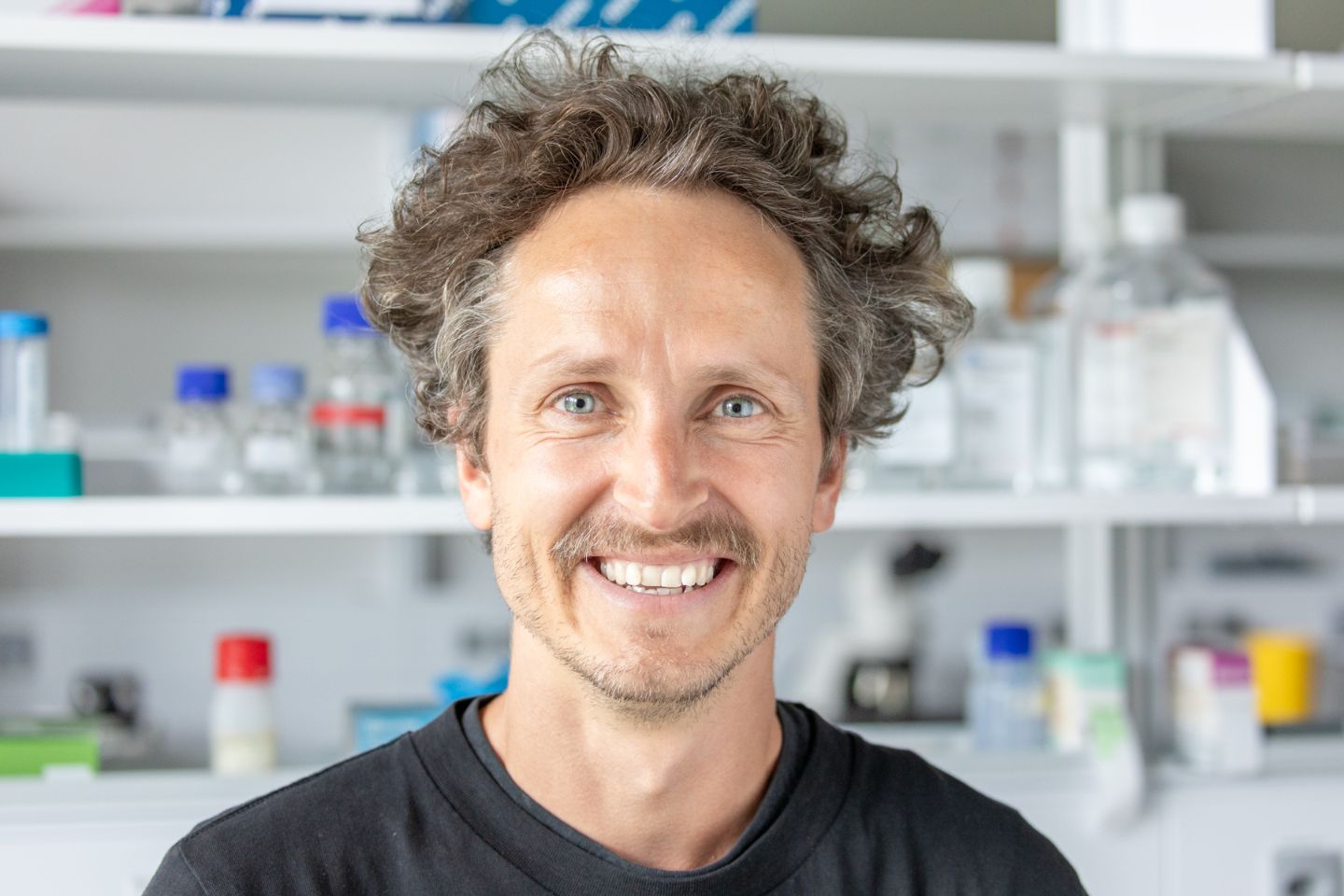
Alumni
- Miriam Rüfenacht , B.Sc.
Master Student - Milad Adibi, Ph.D.
Roche AG - Raza Ali, M.D./Ph.D.
Group Leader, CRUK Cambridge Institute - Maya Barben, Ph.D.
ETH Zürich - Pierre Bost, Ph.D.
Marie Curie Institute - Marcel Burger, Ph.D.
Wissenschaftlicher Mitarbeiter Elemental Analysis, Solvias - Ruben Casanova
- Raúl Catena, Ph.D.
Senior Software Engineer, Leica Geosystems - Stephane Chevrier, Ph.D.
Co-founder, Navignostics AG - Lena Cords, Ph.D.
Postdoctoral Fellow - TU München - Haithem Dakhli, Ph.D.
- Nicolas Damond, Ph.D.
Postdoctoral Fellow - Esther Danenberg, M.Sc.
Research Administrator, CRUK Cambridge Institute - Michelle Daniel, M.Sc.
Navignostics AG - Nadine Dobberstein, M.Sc.
Technician, InterAx Biotech - Nils Eling, Ph.D.
Senior Data Scientist, Novartis - Jana Fischer, Ph.D.
Co-founder, Navignostics AG - Catrina Friedrich, M.Sc.
Master's Student - Charlotte Giesen, Ph.D.
Head, Quality Assurance, Roche - Tobias Hoch, M.Sc.
Research Associate, Empa - Tsuyoshi Hosogane, Ph.D.
Postdoctoral Fellow - Alexandra Huber, B.Sc.
Master's student, ETH Zurich - Hartland Jackson, Ph.D.
Group Leader, Lunenfeld-Tanenbaum Institute - Andrea Jacobs
Co-founder, Navignostics AG - Laura Kütt, Ph.D.
Ph.D. - Pieter Langerhorst, M.Sc.
Ph.D. Student, Radboud Institute - Daria Lazic, M.Sc
Post-doctoral fellow - Xiaokang Lun, Ph.D.
Postdoctoral Fellow, Wyss Institute - Constance Lyon, M.Sc.
Current affiliation unknown - Markus Masek, M.Sc.
Ph.D. Student, University of Zurich - Alaz Özcan, M.Sc.
Ph.D. Student, University of Zurich - Serena Di Palma, Ph.D.
Assistant Professor, Utrecht University - Adhvitha Premanand, B.Tech
- Swetha Raghuraman, M.Sc.
Ph.D. Student, University of Muenster - Luca Räss, M.Sc.
Scientist R&D Automatization, Biognosys AG - Anton Rau, M.Sc.
Software Engineer - Leonor Schubert Santana, M.Sc.
Master's Student - Denis Schapiro, Ph.D.
Postdoctoral Fellow, Broad Institute - Yannik Severin, M.Sc.
Ph.D. Student, ETH Zurich - Sujana Sivapatham, M.Sc.
Research Associate, Immunos Therapeutics - Merrick Strotton, Ph.D.
Prinicpal Scientist, high-plex imaging, UCB - Sandra Tietscher, Ph.D.
Business Development Manager Sensor Innovation at Sensirion - Marco Tognetti, Ph.D.
Senior Scientist, Biognosys AG - Sophie Tritschler, M.Sc.
Ph.D. Student, Helmholtz Z., Muenchen - Eleni Tselempi, M.Sc.
Senior Research Associate, Roche Innovation Centre - James Wade, Ph.D.
QSP Expert, LYO-X GmbH - Johanna Wagner, Ph.D.
Postdoctoral Fellow, NCT Heidelberg - Jonas Windhager, Ph.D.
Bioimage analyst, SciLife Lab - Shuhan Xu, M.Sc.
Ph.D. Student, MPI - Vito Zanotelli, Ph.D.
Data Analytics Consultant, D ONE - Shan Zhao, Ph.D.
Group Leader - Nevena Zivanovic, Ph.D.
Research Scientist, Janssen Pharmaceuticals
Co-founder, Navignostics AG
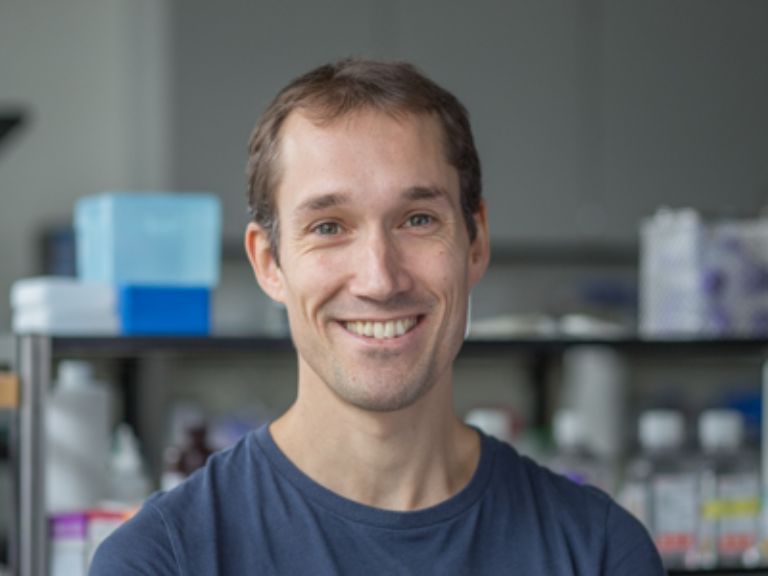
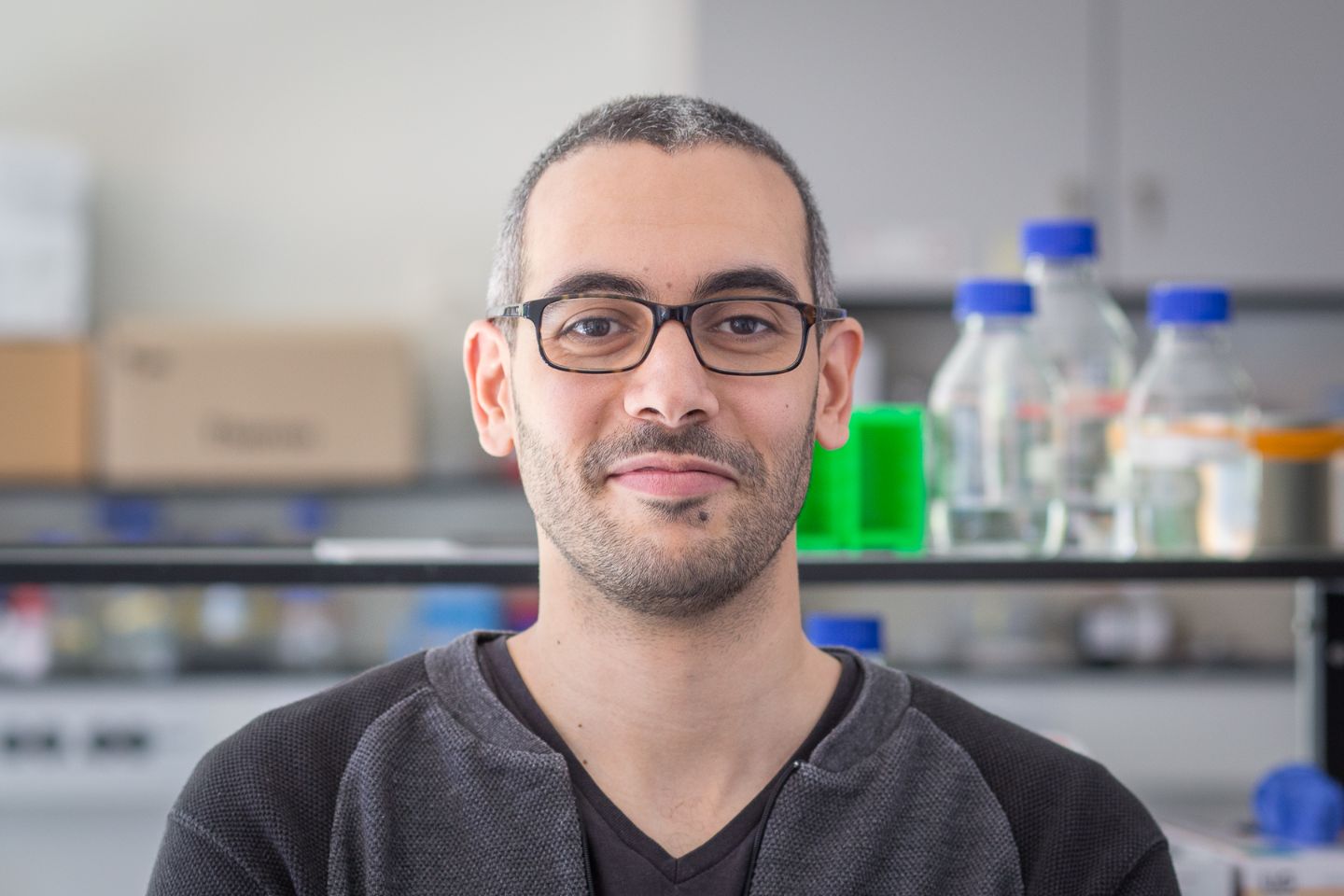
Drug development in breast cancer suffers from a lack of faithful models able to recapitulate breast cancer heterogeneity at the single-cell level. To overcome this limitation, we have generated a patient-derived breast cancer organoid biobank from fresh and frozen breast cancer samples. We are using this model to study the different populations composing the tumor and their response to drug treatments. It is our conviction that the study of the different cellular populations composing breast tumors, and their interactions, will lead to a better understanding of the tumor ecosystem and to the identification of therapies targeting specific cellular populations that could trigger tumor demise.
Postdoctoral Fellow
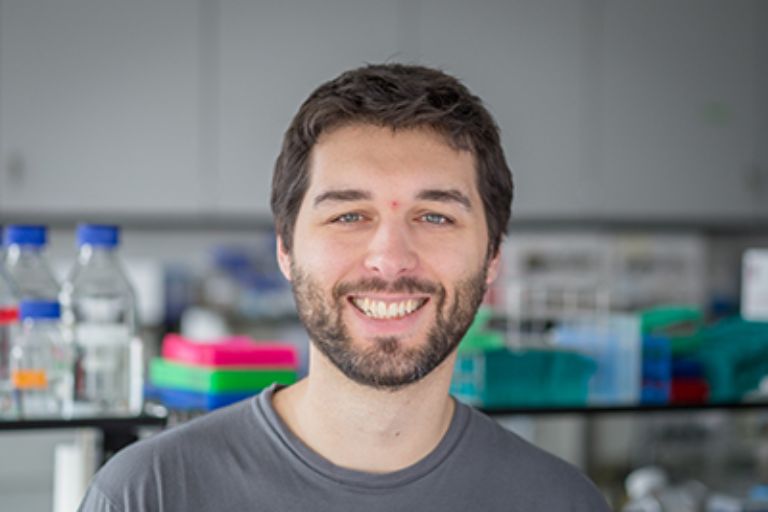
I am combining highly multiplexed imaging, data analysis and experimental biology to characterize type 1 diabetes (T1D) progression and beta cell heterogeneity.
I did my PhD with Prof. Pedro Herrera at the University of Geneva, studying regeneration of insulin-producing beta cells by transdifferentiation of the closely related alpha cells. During my thesis, I used confocal microscopy and lineage tracing in complex transgenic mouse models and primary human pancreatic islets. This work sparked my interest into both T1D research and image analysis of single cells, two elements that are still at the center of my current projects.
In the Bodenmiller lab, I use imaging mass cytometry to analyze pancreas sections of individuals with or at risk for type 1 diabetes. The multiplexing capacity of this technology enables deep phenotyping of beta cells and of infiltrating immune cells, and mapping of their interactions. The goal of this project is to gain a better understanding of T1D development in the pancreas.
In parallel, I'm combining primary human islet culture with imaging mass cytometry to study how beta cell subpopulations respond to external stimuli relevant to T1D. The aim is to identify beta cell subsets that are more sensitive to destructive signals or, conversely, more responsive to regenerative cues. This work is supported by a JDRF postdoctoral fellowship.
Selected Publications
Research Administrator, CRUK Cambridge Institute
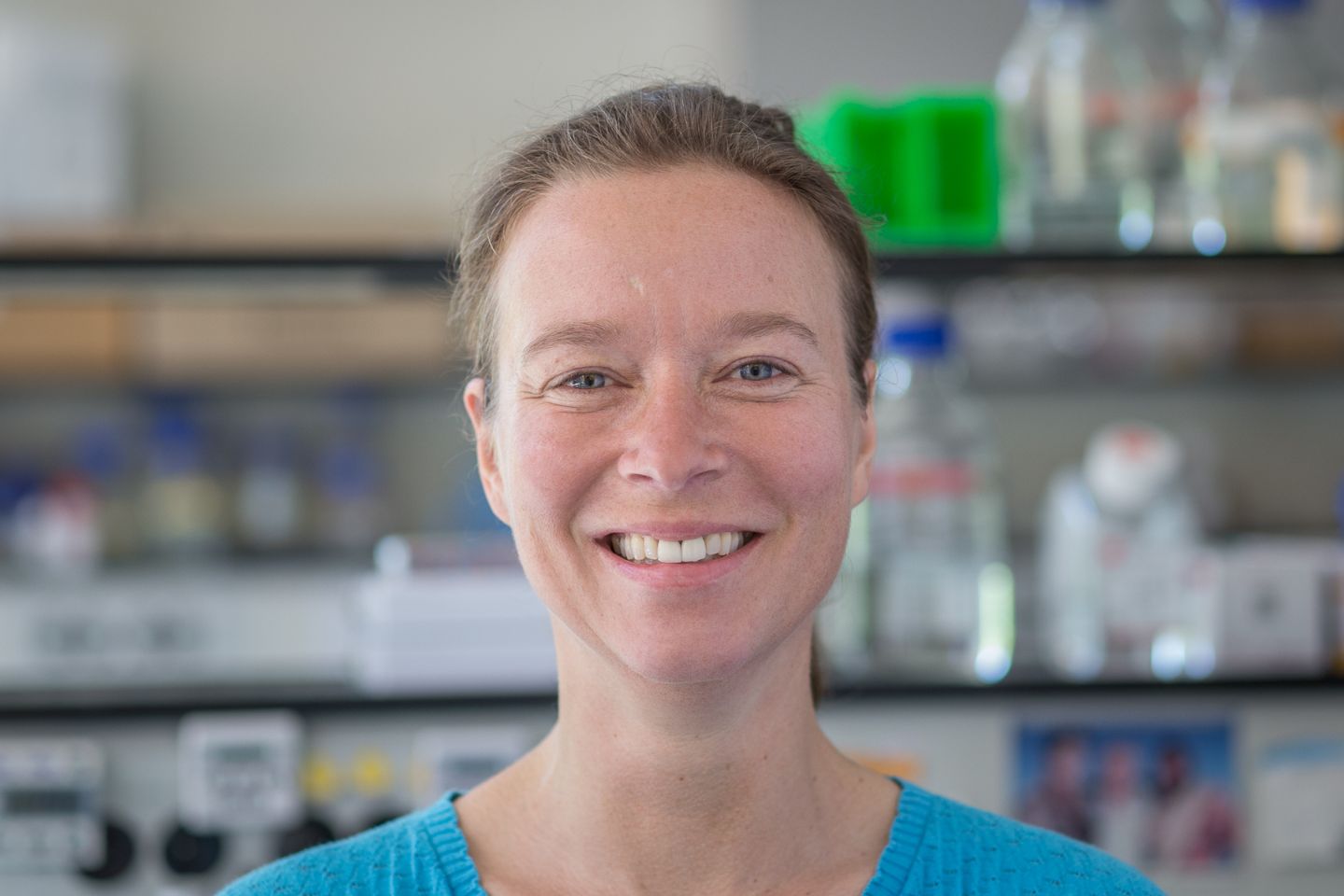
Senior Data Scientist, Novartis
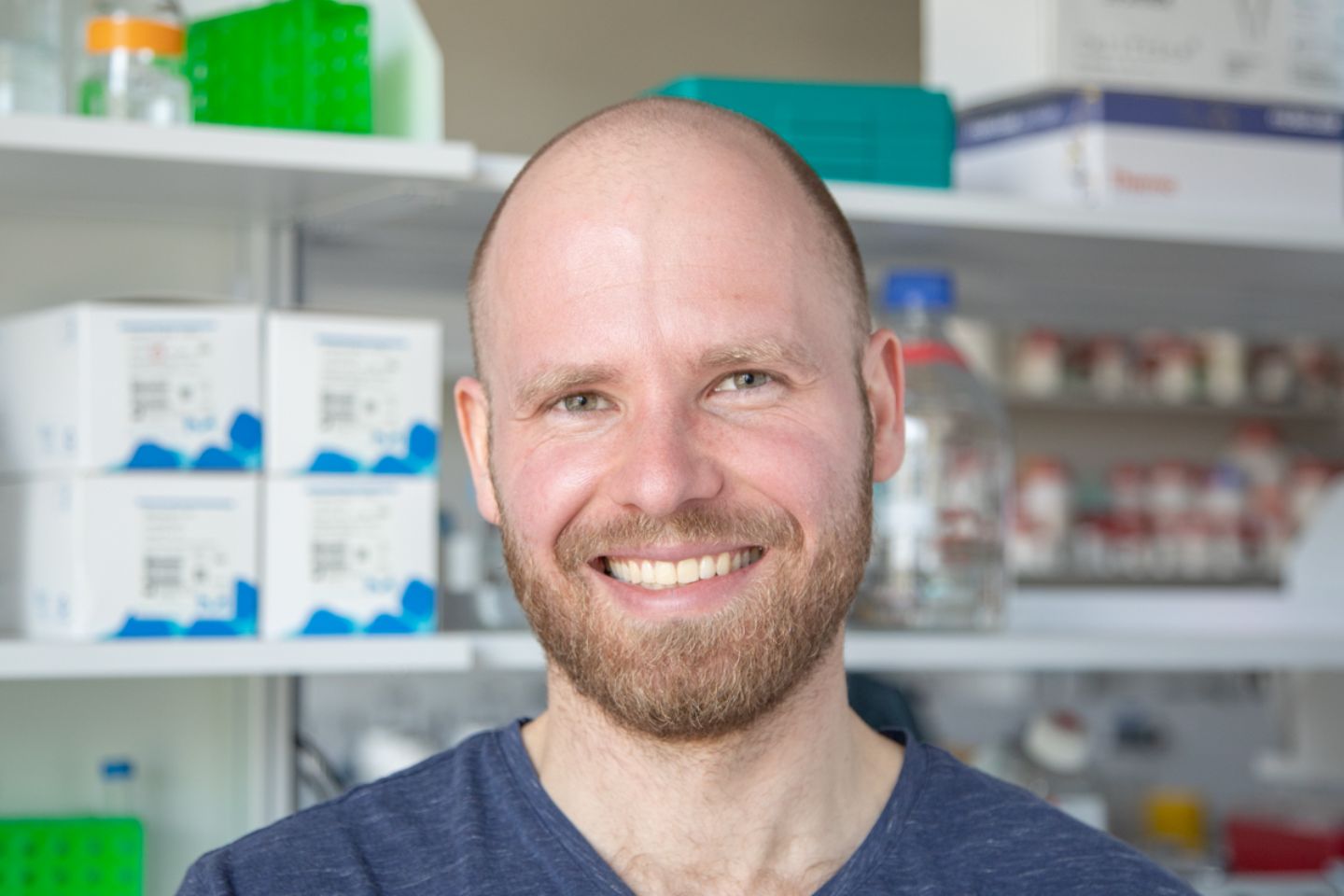
My current research focuses on understanding the molecular changes over breast cancer organoid growth. I'm integrating imaging mass cytometry (IMC) with single-cell based statistical approaches to model spatial heterogeneity in organoids. As part of handling IMC data, I develop software for image and single-cell analysis.
In the past, I have completed my PhD in the Marioni group at the European Bioinformatics Institute and the CRUK Cambridge Institute at the University of Cambridge.
Link to website: nilseling.github.io
Link to Google Scholar: https://scholar.google.com/citations?user=kBIvrFoAAAAJ&hl=de
Link to Github: https://github.com/nilseling
Co-founder, Navignostics AG
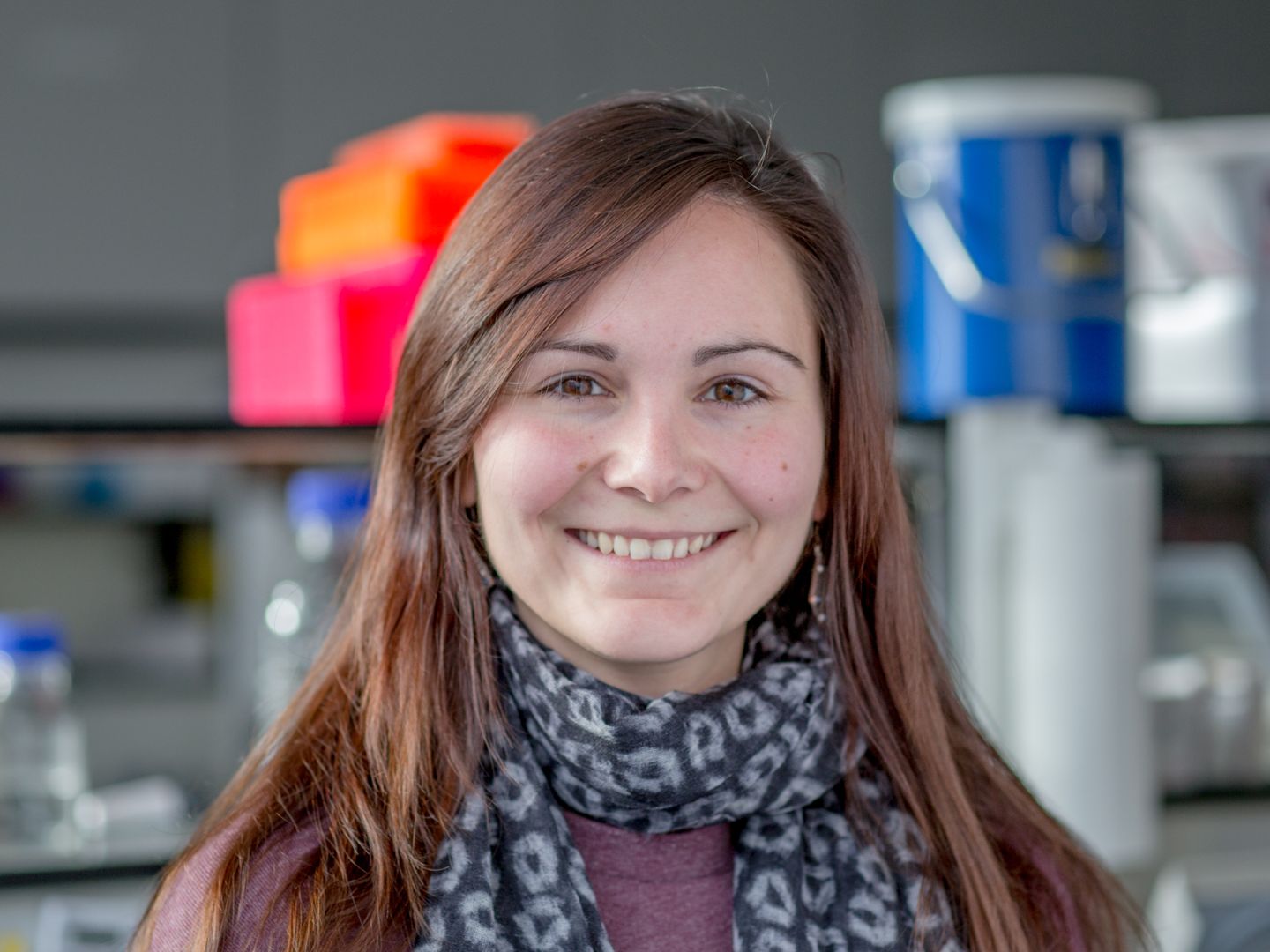
Research Associate, Empa
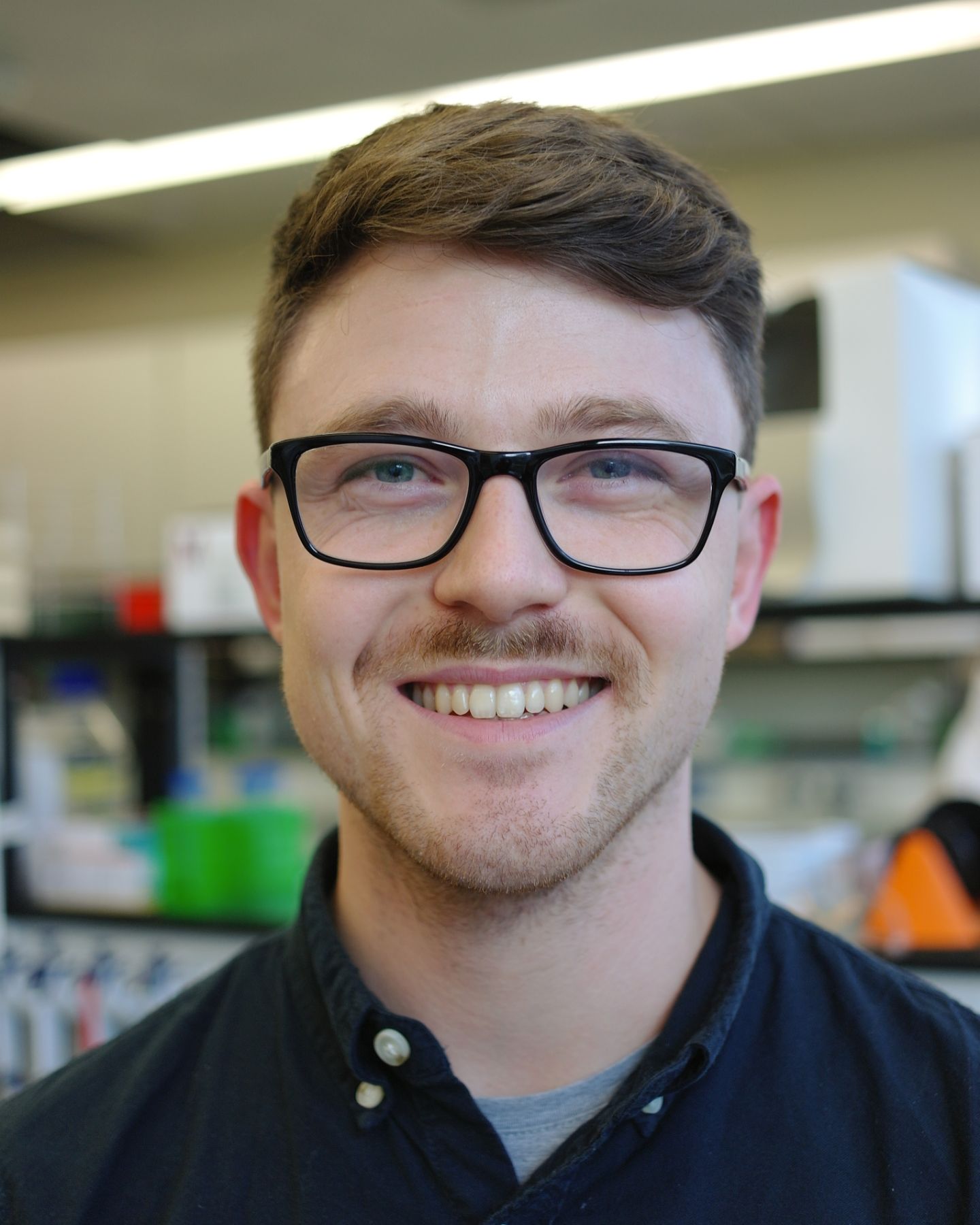
As a research assistant and former Master's student in the lab, I am currently analyzing IMC data with a focus on the immune system and its relation to the protein family of chemokines. I am investigating whether and to what extent the spatial context of chemokine expression can contribute to understanding differences in the immune landscape of patients.
Group Leader, Lunenfeld-Tanenbaum Institute
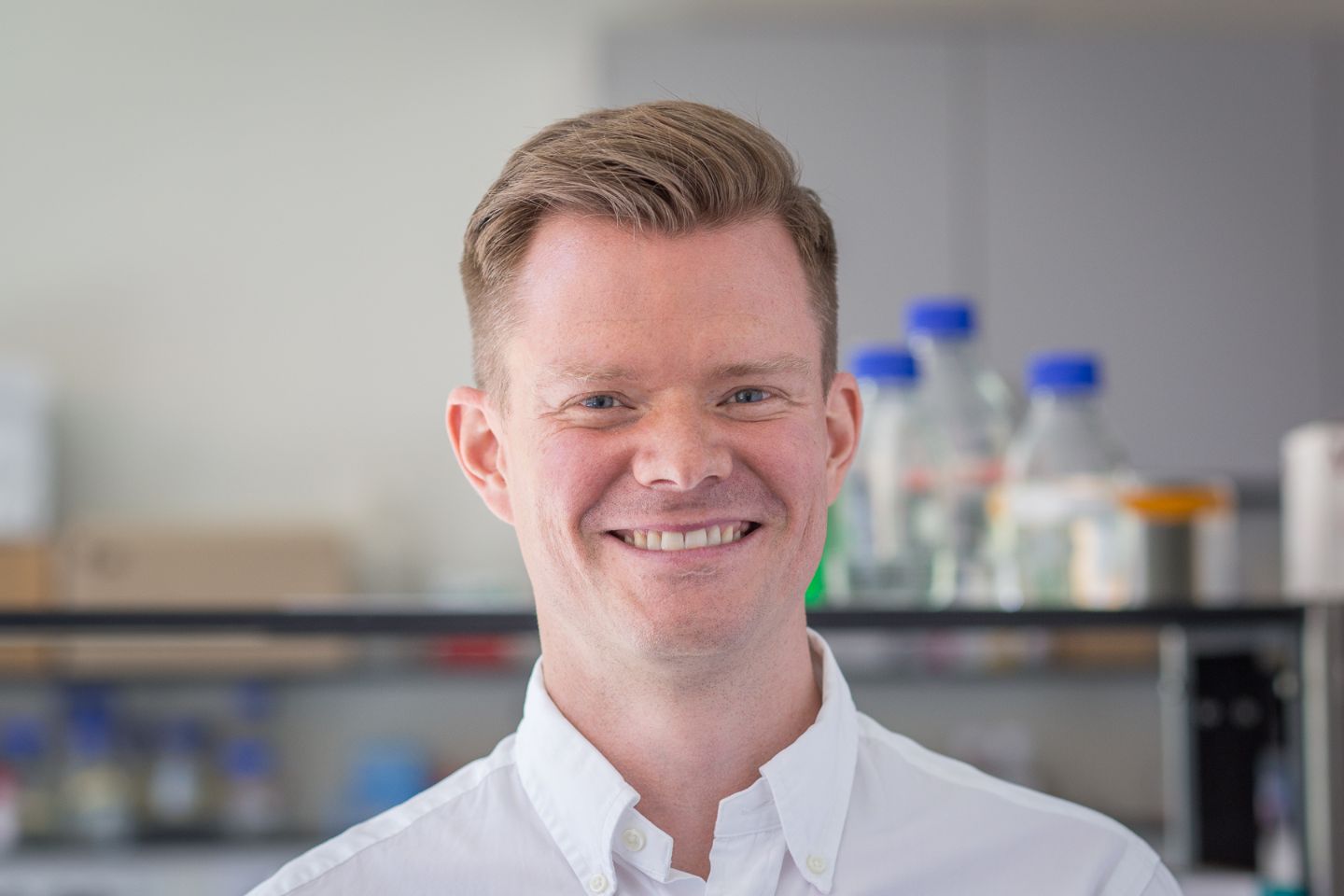
Co-founder, Navignostics AG
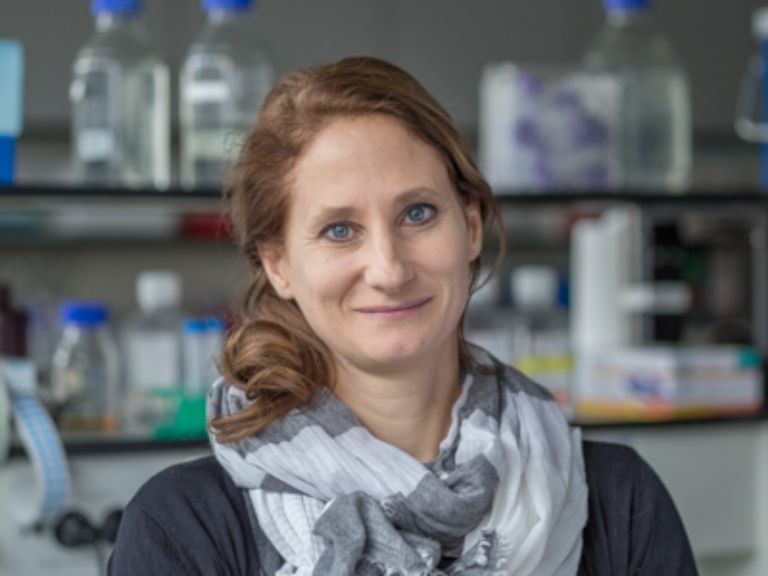
Business Development Manager Sensor Innovation at Sensirion
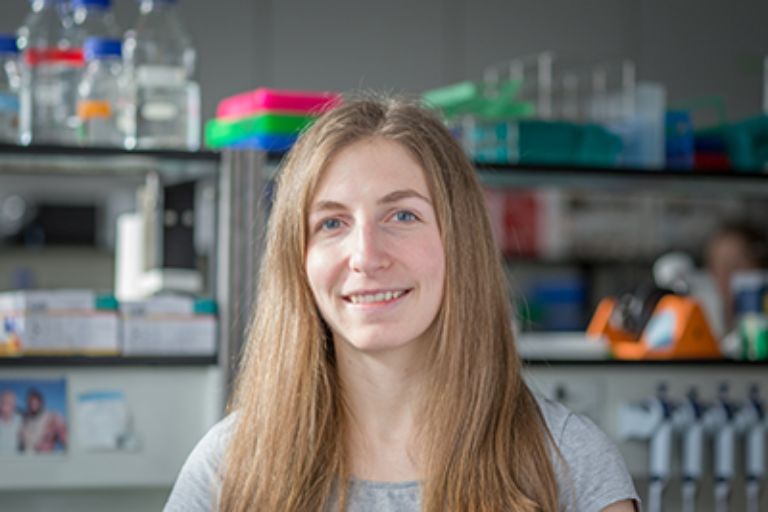
Postdoctoral Fellow, NCT Heidelberg
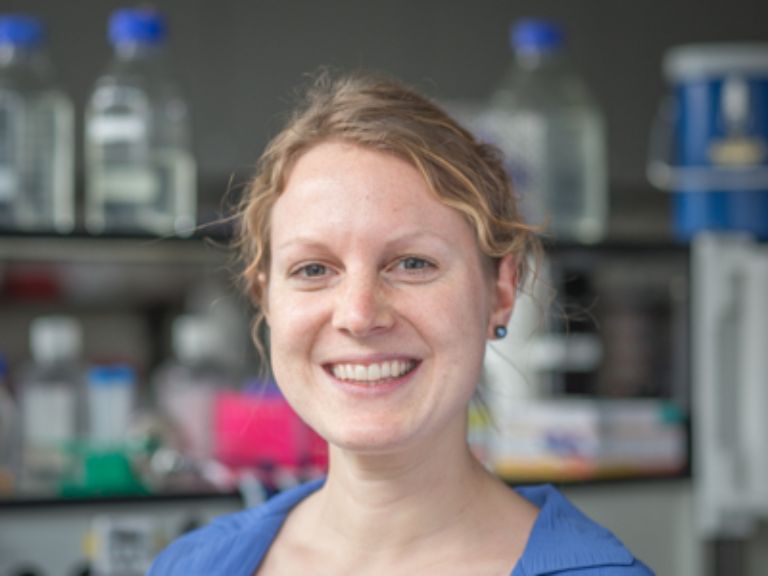
Selected Publications
Data Analytics Consultant, D ONE
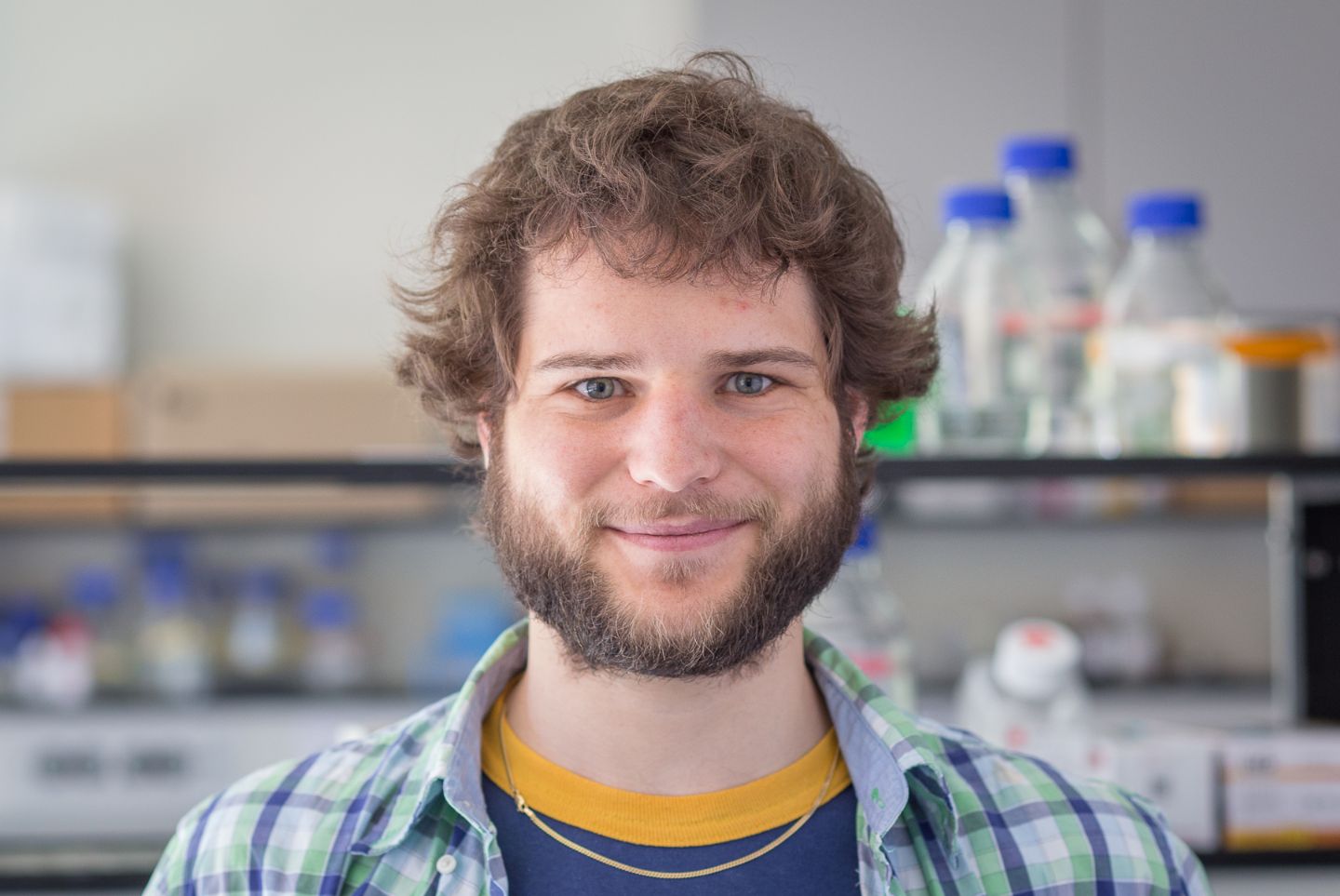
I like to develop high throughput methods together with tailored data analysis approaches to better understand how cells perceive their environment and interact.
Lab Life
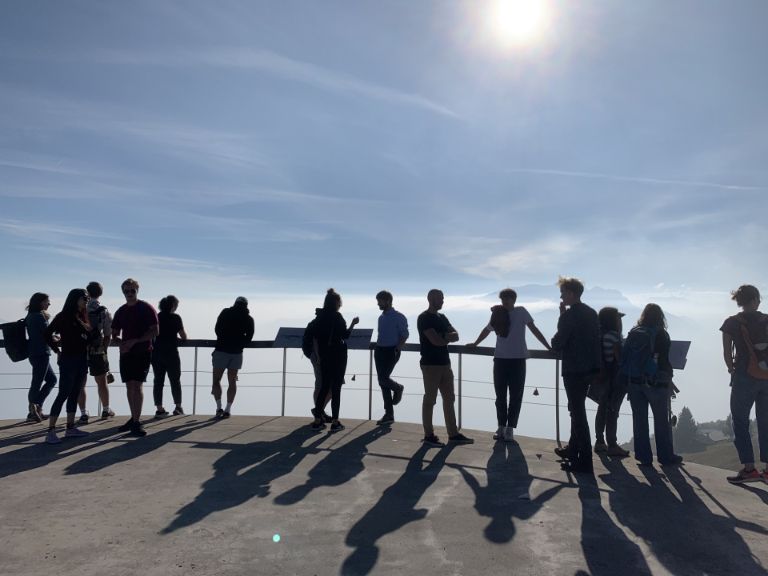
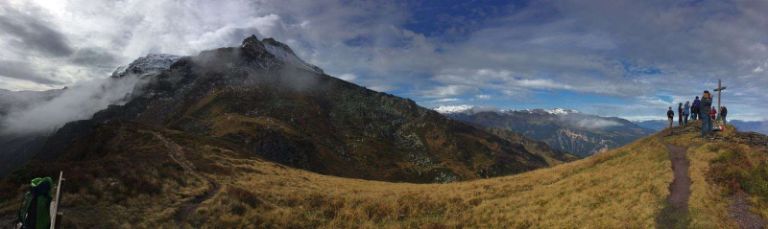
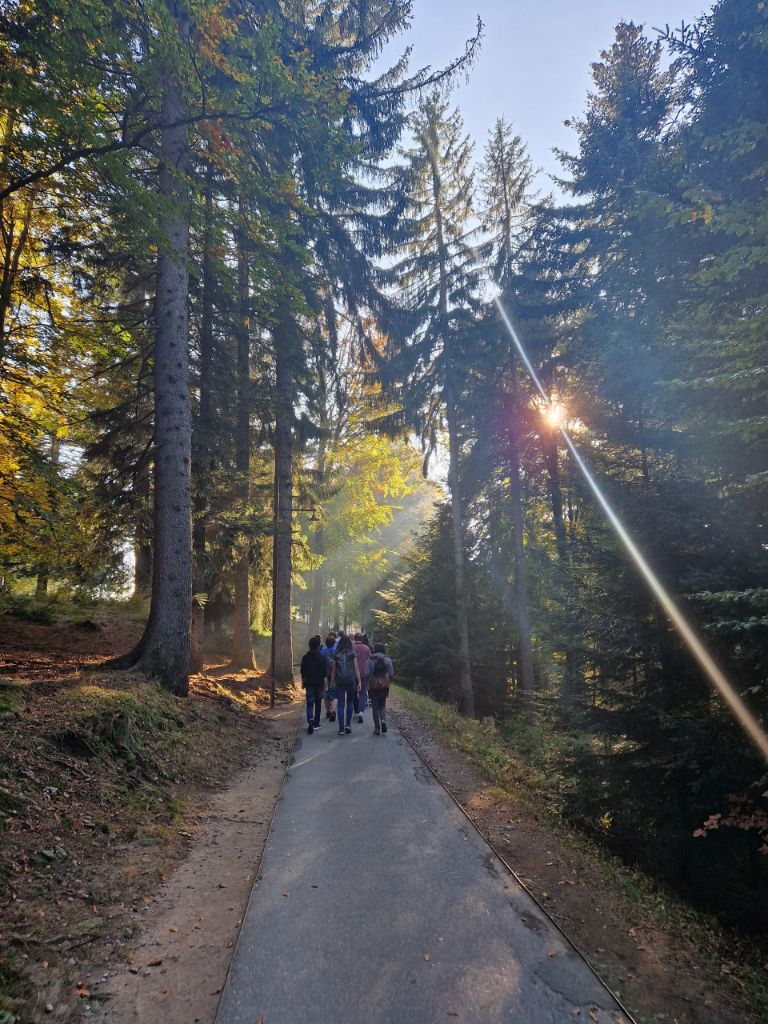
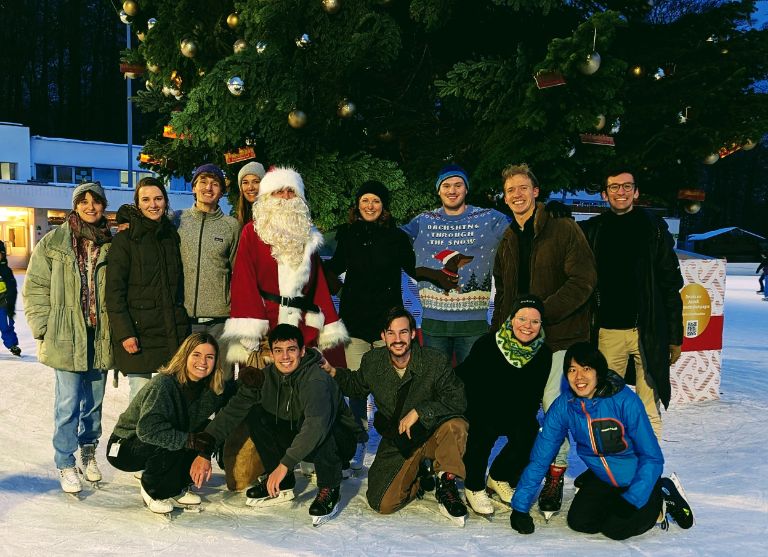
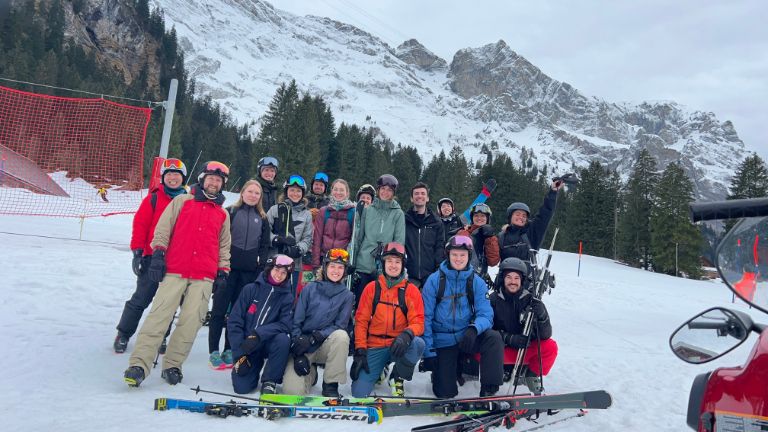
Open Positions
We are always looking for excellent and motivated students/postdocs with a strong background in biological/biochemical/biomedical research or bioinformatics.
Prospective Ph.D. students can apply to our group via the Life Science Zurich Graduate School. Our group is a member of the Molecular Life Sciences, the Systems Biology and the Cancer Biology programs. For computational students, we offer shared Ph.D. positions with bioinformatics research groups.
Post-doctoral candidates should be highly motivated with a passion for science with great interest in quantitative biology, single-cell analysis and systems biomedicine. Our lab and collaborators provide an excellent and vibrant interdisciplinary environment, including systems (cancer) biology, biochemistry, analytical sciences, computer sciences and biomedical research. Candidates should have a demonstrated record of productivity during their Ph.D. studies, including publications in peer-reviewed journals (see DORA).
Applications should be directly sent to Bernd Bodenmiller, including your CV, Publication List and a short paragraph with your scientific interests and what you hope to achieve during your postdoctoral time.
All Publications
Recent Publications
Multiplexed imaging mass cytometry of the chemokine milieus in melanoma characterizes features of the response to immunotherapy
Hoch T, Schulz D, Eling N, Martínez Gómez J, Levesque MP, Bodenmiller B.
Sci Immunol . 2022 Apr;7(70):eabk1692.Multiplexed imaging mass cytometry of the chemokine milieus in melanoma characterizes features of the response to immunotherapy
Hoch T, Schulz D, Eling N, Martínez Gómez J, Levesque MP, Bodenmiller B.
Sci Immunol . 2022 Apr;7(70):eabk1692.Mass cytometric and transcriptomic profiling of epithelial-mesenchymal transitions in human mammary cell lines
Wagner J, Masek M, Jacobs A, Soneson C, Sivapatham S, Damond N, de Souza N, Robinson MD, Bodenmiller B.
Sci Data . 2022 Feb 9;9(1):44.Three-dimensional imaging mass cytometry for highly multiplexed molecular and cellular mapping of tissues and the tumor microenvironment
Kuett L, Catena R, Özcan A, Plüss A, Cancer Grand Challenges IMAXT Consortium, Schraml P, Moch H, de Souza N, Bodenmiller B.
Nat Cancer . 2022 Jan;3(1):122-133.Deciphering the signaling network of breast cancer improves drug sensitivity prediction
Tognetti M, Gabor A, Yang M, Cappelletti V, Windhager J, Rueda OM, Charmpi K, Esmaeilishirazifard E, Bruna, A, de Souza N, Caldas C, Beyer A, Picotti P, Saez-Rodriguez J, Bodenmiller B.
Cell Syst. 2021 May 19;12(5):401-418.e12.A quantitative analysis of the interplay of environment, neighborhood, and cell state in 3D spheroids
Zanotelli VRT, Leutenegger M, Lun XK, Georgi F, de Souza N, Bodenmiller B.
Mol Syst Biol. 2020 Dec;16(12):e9798.A distinct innate immune signature marks progression from mild to severe COVID-19
Chevrier S, Zurbuchen Y, Cervia C, Adamo S, Raeber ME, de Souza N, Sivapatham S, Jacobs A, Bachli E, Rudiger A, Stüssi-Helbling M, Huber LC, Schaer DJ, Nilsson J, Boyman O, Bodenmiller B.
Cell Rep Med. 2020 Dec 26;2(1):100166.Mechanistic Model of Signaling Dynamics Across an Epithelial Mesenchymal Transition
Wade JD, Lun XK, Zivanovic N, Voit EO, Bodenmiller B.
Front Physiol. 2020 Nov 30;11:579117.Profiling cell signaling networks at single-cell resolution
Lun XK, Bodenmiller B.
Mol Cell Proteomics. 2020 May;19(5):744-756.The single-cell pathology landscape of breast cancer.
Jackson HW, Fischer JR, Zanotelli VRT, Ali HR, Mechera R, Soysal SD, Moch H, Muenst S, Varga Z, Weber WP, Bodenmiller B.
Nature 2020 Feb;578(7796):615-620.Imaging mass cytometry and multiplatform genomics defines the phenogenomic landscape of breast cancer.
HR Ali, Jackson HW, Zanotelli VRT, Danenberg E, Fischer JR, Bardwell H, Provenzano E, CRUK IMAXT Grand Challenge Team, Rueda OM, Chin SF, Aparico S, Caldas C, Bodenmiller B.
Nature Cancer 1, 163–175 (2020)Analysis of the human kinome and phosphatome by mass cytometry reveals overexpression-induced effects on cancer-related signaling
Lun XK, Szklarczyk D, Gábor A, Dobberstein N, Zanotelli VRT, Saez-Rodriguez J, von Mering C, Bodenmiller B.
Mol Cell. 2019 Jun 6;74(5):1086-1102.A single-cell atlas of the tumor and immune ecosystem of human breast cancer
Wagner J, Rapsomaniki MA, Chevrier S, Anzeneder T, Langwieder C, Dykgers A, Rees M, Ramaswamy A, Muenst S, Soysal SD, Jacobs A, Windhager J, Silina K, van den Broek M, Dedes KJ, Rodríguez Martínez M, Weber WP, Bodenmiller B.
Cell. 2019 May 16;177(5):1330-1345.e18.
Selected Publications
The single-cell pathology landscape of breast cancer.
Jackson HW, Fischer JR, Zanotelli VRT, Ali HR, Mechera R, Soysal SD, Moch H, Muenst S, Varga Z, Weber WP, Bodenmiller B.
Nature 2020 Feb;578(7796):615-620.A single-cell atlas of the tumor and immune ecosystem of human breast cancer
Wagner J, Rapsomaniki MA, Chevrier S, Anzeneder T, Langwieder C, Dykgers A, Rees M, Ramaswamy A, Muenst S, Soysal SD, Jacobs A, Windhager J, Silina K, van den Broek M, Dedes KJ, Rodríguez Martínez M, Weber WP, Bodenmiller B.
Cell. 2019 May 16;177(5):1330-1345.e18.Analysis of the human kinome and phosphatome by mass cytometry reveals overexpression-induced effects on cancer-related signaling
Lun XK, Szklarczyk D, Gábor A, Dobberstein N, Zanotelli VRT, Saez-Rodriguez J, von Mering C, Bodenmiller B.
Mol Cell. 2019 Jun 6;74(5):1086-1102.A map of human type I diabetes progression by imaging mass cytometry.
Damond N, Engler S, Zanotelli VRT, Schapiro D, Wasserfall CH, Kusmartseva I, Nick HS, Thorel F, Herrera PL, Atkinson MA, Bodenmiller B.
Cell Metabolism 29(3): 755-768.E5Compensation of signal spillover in suspension and imaging mass cytometry.
Chevrier S, Crowell HL, Zanotelli VRT, Engler S, Robinson MD, Bodenmiller B.
Cell Systems 6(5), 612-620 (2018).Simultaneous multiplexed imaging of mRNA and proteins with subcellular resolution in breast cancer tissue samples by mass cytometry
Schulz D, Zanotelli VRT, Fischer JR, Schapiro D, Engler S, Lun XK, Jackson, HW, Bodenmiller B.
Cell Systems 6(1), 25 - 36 (2018).HistoCAT: analysis of cell phenotypes and interactions in multiplex image cytometry data.
Schapiro D, Jackson HW, Raghuraman S, Fischer JR, Zanotelli VRT, Schulz D, Giesen C, Catena R, Varga Z, Bodenmiller B.
Nature Methods 14, 873–876 (2017).An immune atlas of clear cell renal cell carcinoma
Chevrier S, Levine JH, Zanotelli VRT, Silina K, Schulz D, Bacac M, Ries CH, Ailles L, Jewett MAS, Moch H, van den Broek M, Beisel C, Stadler MB, Gedye C, Reis B, Pe'er D, Bodenmiller B.
Cell 169(4), 736 - 749 (2017).Influence of node abundance on signaling network state and dynamics analyzed by mass cytometry.
Lun XK, Zanotelli VRT, Wade JW, Schapiro D, Tognetti M, Dobberstein N, Bodenmiller B.
Nature Biotechnology 35, 164–172 (2017).Highly multiplexed imaging of tumor tissues with subcellular resolution by mass cytometry.
Giesen C, Wang HAO, Schapiro D, Zivanovic N, Jacobs A, Hattendorf B, Schueffler PJ, Grolimund D, Buhmann JM, Brandt S, Varva Z, Wild PJ, Guenther D, Bodenmiller B.
Nature Methods 11, 417–422 (2014).
Teaching
We provide a range of master and semester projects for motivated students with interest in biological/biochemical/biomedical research or bioinformatics. Applications should be sent directly to Bernd Bodenmiller, including your CV and a short paragraph with your scientific interests.
Alternatively, for hands-on experience in the Bodenmiller lab, we offer the block courses BME331 & BME330 in the Spring and Autumn semester respectively.
For details of provided lectures, please see course descriptions at UZH and ETHZ.
Master’s Thesis or Other Credit-Earning Assignment in Deep Learning for Oncology
The Bodenmiller Lab at UZH/ETH is seeking talented and motivated Master’s students with expertise in machine learning to join our team. We offer exciting opportunities to work on cutting-edge deep learning projects focused on tumor-tissue image analysis as part of a Master’s thesis or a credit-earning assignment (e.g. unpaid internship, lab rotation, semester project).
About the Projects
Imaging Mass Cytometry (IMC) is an advanced imaging technology that combines mass spectrometry with microscopy to generate highly multiplexed spatial data at single-cell resolution. Unlike traditional imaging methods that rely on fluorescent markers, IMC uses metal-tagged antibodies to detect dozens of proteins simultaneously within a tissue sample. This enables researchers to study complex cellular environments—such as tumors or immune tissues—with exceptional detail. Currently, the standard approach for analyzing IMC images of tumor tissue involves cell segmentation, where individual cells are identified and treated as the smallest units of analysis. However, this method introduces potential biases and overlooks valuable contextual information in the surrounding tissue. To overcome these limitations, we are developing deep learning approaches that analyze IMC data directly at the pixel level, enabling a more comprehensive and unbiased understanding of tissue architecture and cellular interactions. Potential Projects:
- Optimization of Vision Transformer (ViT) architectures to improve IMC image data analysis
- Enhancing explainability of pixel-based IMC models to better understand deep learning predictions
- Validating pixel-based IMC models by comparing them to single-cell analysis techniques
These projects offer a unique opportunity to apply and develop advanced AI models, work with high-dimensional biological data, and contribute to impactful biomedical research.
What We Offer
- A dynamic and interdisciplinary research environment at the intersection of machine learning and biological sciences
- The chance to work closely with leading researchers in computational biology and biomedical data science
- Hands-on experience applying deep learning to biomedical research
- Flexible start dates
Who Should Apply?
We are looking for students who:
- Are proficient in Python and PyTorch
- Are self-reliant and have strong problem-solving skills
- Have an interest in computer vision and cancer biology
Nice to have:
- A background in computer science, engineering, computational biology or bioinformatics
- Software or machine learning engineering experience
- Experience with high-performance computing environments
- Experience with workflow management systems
How to Apply
Interested students should submit the following documents:
- CV (max. 2 pages)
- A short motivation letter (max. 1)
- Transcript of records (we will focus primarily on relevant coursework rather than overall grades)
- Optional: A link to a GitHub repository showcasing a relevant application
Applications should be sent in ONE email addressed to both
Contact
Department of Quantitative Biomedicine
University of Zurich
Winterthurerstrasse 190
CH-8057 Zurich
Switzerland
We are not easy to find, download our campus map.
Building / Room: Y38-M
phone: +41 44 635 48 25
fax: +41 44 635 68 79
Inst. f. Molecular Health Sciences
ETH Zurich
Otto-Stern-Weg 7
CH-8093 Zürich
Switzerland
Building / Room: HPL H 16.2
phone: +41 44 633 29 20